This tutorial explains how to write code for boostrapping mediation analysis in R from scratch. Mediation analysis is often used in academic research to understand the underlying mechanism. Often, you also need to report a bootstrapping confidence interval for the indirect effect.
Steps of Bootstrapping Mediation Analysis in R
The following shows the steps.
- (1) sample a data to estimate path a, and path b, and them time a and b together to get one indirect effect.
- (2) Use boot() to repeat 5000 times. Thus, you get 5000 indirect effects.
- (3) Use the output from boot() to calculate the confidence intervals.
The following is the flow chart of doing bootstrapping mediation analysis in R from scratch.

R code from Scratch for Bootstrapping Mediation
The following is the complete R code of running bootstrapping mediation analysis in R.
Normal_Mediation<-function(data_used,i) { data_temp=data_used[i,] # a path result_a_temp<-lm(M~X, data = data_temp)$coefficients names(result_a_temp) <- NULL a_0_temp<-result_a_temp[1] a_1_temp<-result_a_temp[2] # b path result_b_temp<-lm(Y~M+X, data = data_temp)$coefficients names(result_b_temp) <- NULL b_0_temp<-result_b_temp[1] b_1_temp<-result_b_temp[2] c_1_apostrophe_temp<-result_b_temp[3] #calculating the indirect effect indirect_temp<-a_1_temp*b_1_temp return(indirect_temp) } #read data from GitHub Mediation_data <- read.csv('https://raw.githubusercontent.com/TidyPython/Mediation_analysis/main/mediation_hypothetical_data.csv') set.seed(123) library(boot) boot_mediation <- boot(Mediation_data, Normal_Mediation, R=5000) boot_mediation boot.ci(boot.out = boot_mediation, type = c("norm", "basic", "perc", "bca"))
The following is the output. Thus, we can see that the indirect effect is 0.097. The 95% confidence interval based on normal distribution assumption is ( 0.0377, 0.1570 ). The 95% confidence interval using percentile is ( 0.0379, 0.1559 ).
Call: boot(data = Mediation_data, statistic = Normal_Mediation, R = 5000) Bootstrap Statistics : original bias std. error t1* 0.09748237 0.0001266305 0.03044034 BOOTSTRAP CONFIDENCE INTERVAL CALCULATIONS Based on 5000 bootstrap replicates CALL : boot.ci(boot.out = boot_mediation, type = c("norm", "basic", "perc", "bca")) Intervals : Level Normal Basic 95% ( 0.0377, 0.1570 ) ( 0.0391, 0.1571 ) Level Percentile BCa 95% ( 0.0379, 0.1559 ) ( 0.0360, 0.1538 ) Calculations and Intervals on Original Scale
Plot bootstrapping mediation analysis in R
We can also check the distribution of all the indirect effects, which has 5000.
plot(boot_mediation)
The following is the plot of the indirect effect from bootstrapping mediation analysis.
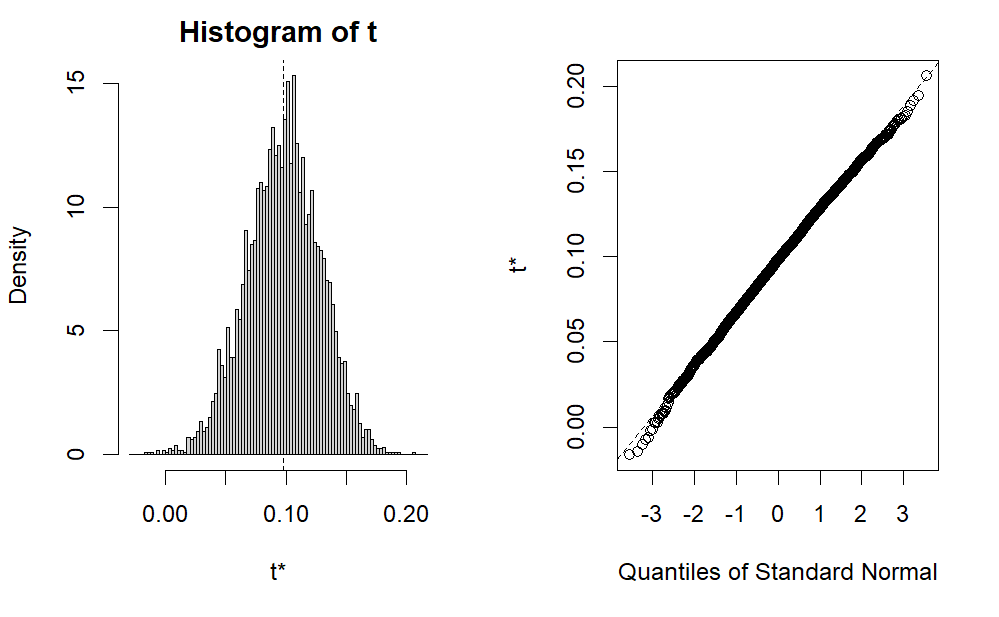
Standard error and standard deviation of bootstrapping
We can also calculate the standard error of the indirect effect. Note that, based on wikipedia, standard error (SE) of a statistic (usually an estimate of a parameter) is the standard deviation of its sampling distribution or an estimate of that standard deviation. Thus, we can just calculate the standard deviation of it.
sd(boot_mediation$t)
The following is the output. It is consistent the output shown above. That is, it is the same as the standard error shown in the “Bootstrap Statistics.”
[1] 0.03044034
Confidence interval functions for bootstrapping from scratch
We can also calcuate the Confidence Interval using our own functions. The following calculates both the normal standard distribution and percentile ones.
# this is based on normal distribution print(boot_mediation$t0 + c(-1, 1) * 1.96 * sd(boot_mediation$t)) # this is based on percentile print(quantile(boot_mediation$t,c(0.025,0.975),type = 6))
The following is the output, which is consitent (but not exactly the same) with the ones shown above.
# this is based on normal distribution [1] 0.03781931 0.15714543 # this is based on percentile 2.5% 97.5% 0.0378975 0.1558657
Reference
The following UCLA page provides a quick introduction of boot() function.
The following is a detailed tutorial about boot() function. It is long but useful.
The following provides a quick introduction of mediation analysis using bootstrapping.
Further Reading
You might also find the following tutorials on regression and Johnson Neyman in R useful.